
The ME-Model is a microbial growth model that computes the optimal cellular state for growth in a given steady-state environment. The WCM and genome-scale optimality models thus have different capabilities and will find use to predict and explain different biological phenomena. The WCM does not have this ability owing to the disparate mathematical formalisms it employs. While the model described by Karr et al is a major advance toward whole-cell computation, many practical applications rely on the ability to compute optimal phenotypic states. The WCM integrates many more cellular processes and can be used to simulate dynamic cellular states however, it depends on detailed molecular measurements of an initial state (e.g., growth rate, biomass composition, and gene expression). On the heels of these developments, a whole-cell model (WCM) of the human pathogen Mycoplasma genitalium appeared ( Karr et al, 2012). Recently, M-Models have been extended to include the process of gene expression (termed as ME-Models) ( Lerman et al, 2012 Thiele et al, 2012), opening up completely new vistas in the development of microbial systems biology. Genome-scale optimality models of metabolism (termed as M-Models) have made much progress in recent years as they require only basic knowledge of reaction stoichiometry, are genome-scale in scope, and have fairly accurate predictive power.
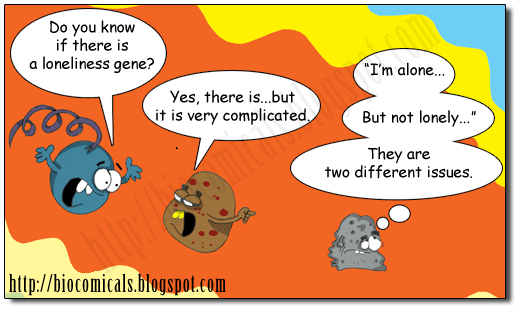
The type of modeling formalism employed is influenced by all of these distinguishing characteristics ( Machado et al, 2011). What distinguish these models from each other are the underlying assumptions they make, the input data they require, and the scope and precision of their predictions ( Selinger et al, 2003). For this reason, modeling whole biological systems (or subsystems) has received increased attention.Ī number of modeling approaches have been developed to predict systems-level phenotypes. However, reductionist viewpoints, by definition, do not provide a coherent understanding of whole cell functions. Reductionist approaches to biology have produced ‘parts lists’, and successfully identified key concepts (e.g., central dogma) and specific chemical interactions and transformations (e.g., metabolic reactions) fundamental to life. Understanding the genotype–phenotype relationship demands vantage points at multiple scales, ranging from the molecular to the cellular. Historically, and still for most phenotypic traits, this relationship is described through qualitative arguments based on observations or through statistical correlations. The genotype–phenotype relationship is fundamental to biology. Our results unify many existing principles developed to describe bacterial growth. We formalize these constraints and apply the principle of growth optimization to enable the accurate prediction of multi-scale phenotypes, ranging from coarse-grained (growth rate, nutrient uptake, by-product secretion) to fine-grained (metabolic fluxes, gene expression levels). Metabolism and gene expression are interdependent processes that affect and constrain each other. The model computes ∼80% of the functional proteome (by mass), which is used by the cell to support growth under a given condition. Here, we construct an ME-Model for Escherichia coli-a genome-scale model that seamlessly integrates metabolic and gene product expression pathways. Such a model must be predictive of events at the molecular scale and capable of explaining the high-level behavior of the cell as a whole. Growth requirements are well-characterized experimentally for many microbes however, we lack a unified model for cellular growth.
